Technology Report
Executive Summary
- Generative artificial intelligence (AI) should strengthen cybersecurity, particularly in threat identification, although it’s unlikely to lead to full automation anytime soon.
- Bad actors are also exploring generative AI’s potential to aid cyberattacks through innovations such as self-evolving malware.
- Through a range of moves today, both buyers and providers of cybersecurity services can take advantage of the new technology while remaining protected.
Este artigo faz parte do Relatório de Fusões e Aquisições de 2023 da Bain.
Only months after its public breakthrough, generative AI has shown the potential to transform cybersecurity products and operations. After the launch of ChatGPT and other products powered by large language models (LLMs), the cybersecurity industry is planning for generative AI to become a key tool. And that’s despite the launch challenge generative AI faces in cybersecurity—namely, the sensitive and siloed nature of security data, which makes it hard to get high-quality, comprehensive datasets to train and update an LLM model.
So far, threat identification is the hot spot. When we analyzed cybersecurity companies that are using generative AI, we found that all were using it at the identification stage of the SANS Institute’s well-known incident response framework—the biggest uptake in any of the six SANS stages (preparation, identification, containment, eradication, recovery, and lessons learned). That fits our assessment that threat identification holds the greatest potential for generative AI to improve cybersecurity (see Figure 1). Generative AI is already helping analysts spot an attack faster, then better assess its scale and potential impact. For instance, it can help analysts more efficiently filter incident alerts, rejecting false positives. Generative AI’s ability to detect and hunt threats will only get more dynamic and automated.
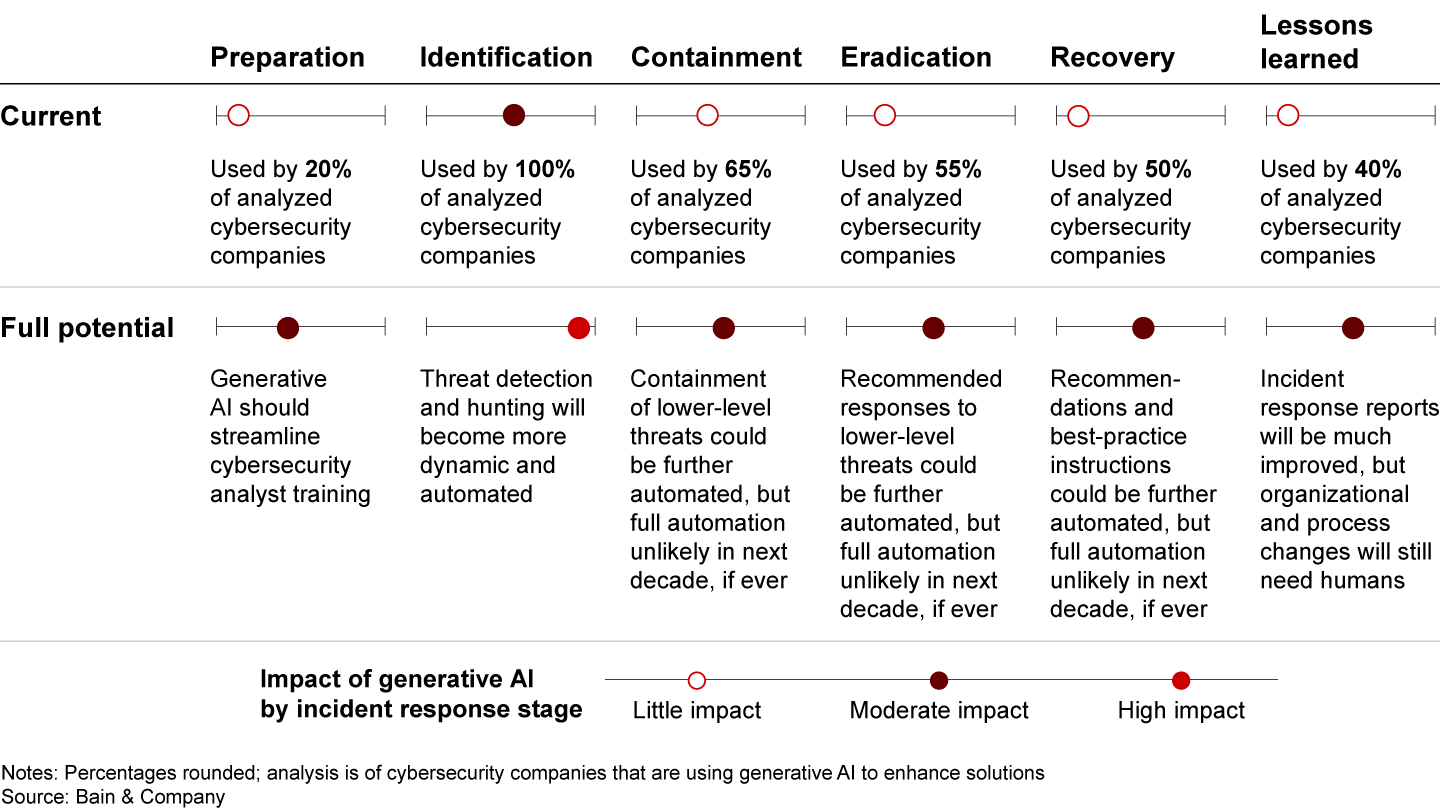

For the containment, eradication, and recovery stages of the SANS framework, adoption rates vary from about one-half to two-thirds of the cybersecurity companies we analyzed, with containment most advanced. In these stages, generative AI is already narrowing knowledge gaps by providing analysts with remedy and recovery instructions based on proven tactics from past incidents. While there will be more gains through automation of containment, eradication, and recovery plans, full automation is unlikely over the next 5 to 10 years, if at all. The longer-term impact of generative AI in these areas is likely to be moderate and will likely always need some human supervision.
Generative AI is also being used in the lessons-learned stage, where it can automate the creation of incident response reports, improving internal communication. Crucially, the reports can be reincorporated into the model, improving defenses. For example, Google’s Security AI Workbench, powered by the Sec-PaLM 2 LLM, converts raw data from recent attacks into machine-readable and human-readable threat intelligence that can accelerate responses (under human supervision). But while the quality of generative AI–powered incident response reports should keep improving, human involvement is still likely to remain necessary.
A double-edged sword
Of course, generative AI can also be used as a cyberattacker's tool, giving them similar capabilities as defenders. For example, less experienced attackers can use it to create more enticing emails or more realistic deepfake videos, recordings, and images to send to phishing targets. Generative AI also allows bad actors to easily rewrite a known attack code to be just different enough to avoid detection.
Generative AI has certainly become a trending topic for malicious actors. Mentions of generative AI on the dark web proliferated in 2023 (see Figure 2). It’s common to see hackers boasting that they’re using ChatGPT. One hacker posted that he was able to use generative AI to recreate malware strains from research publications, such as a Python-based stealer that can search and retrieve common file types (.docx, PDF, images) across a system.
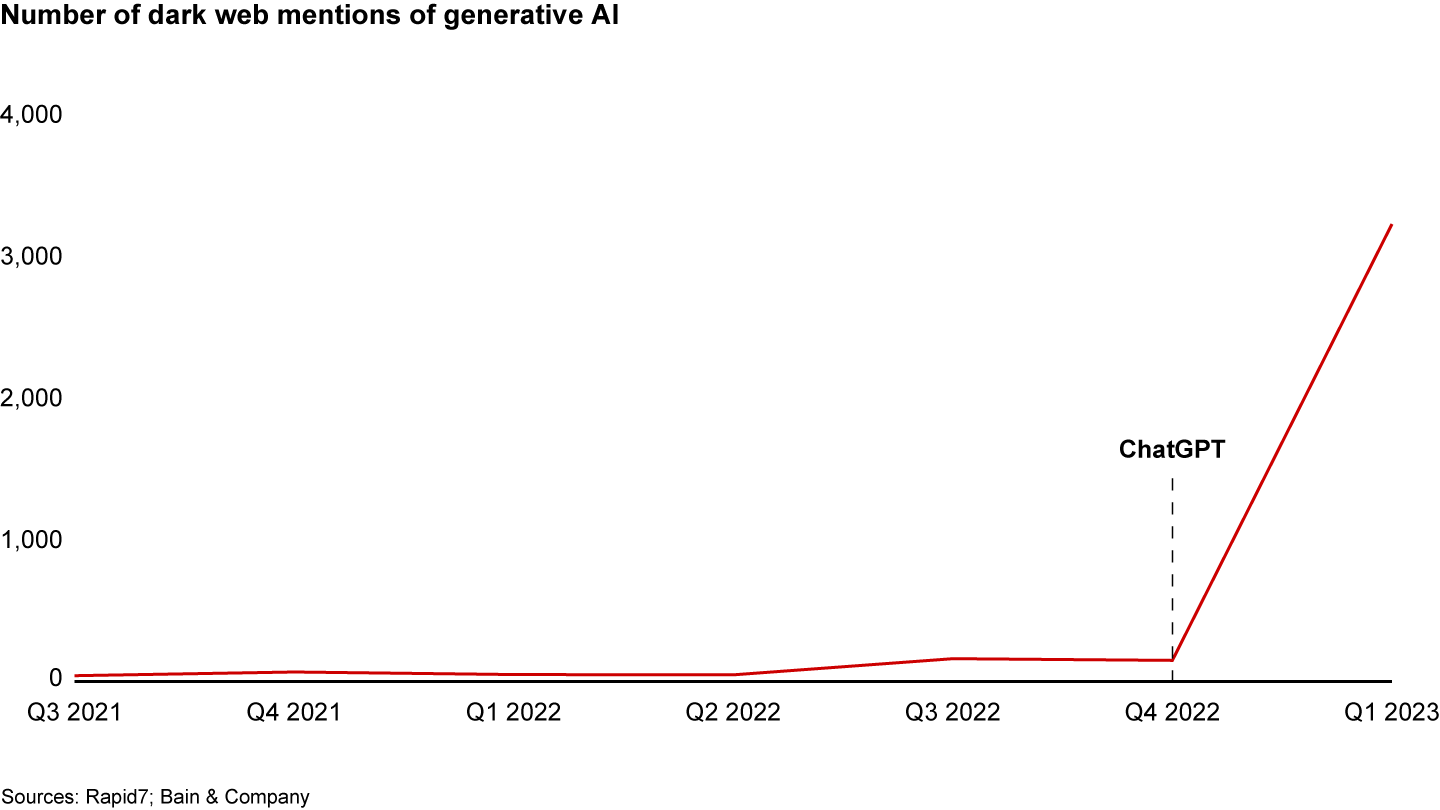

The threat from bad actors will only increase as they use generative AI to standardize and update their tactics, techniques, and procedures. Generative AI–assisted dangers include strains of malware that self-evolve, creating variations to attack a specific target with a unique technique, payload, and polymorphic code that’s undetectable by existing security measures. Only the most agile cybersecurity operations will stay ahead.
Actions to take now
Corporate leaders should:
- understand that generative AI won’t rid cybersecurity of its operational and technical complexities;
- make generative AI and cybersecurity a recurring agenda item for board and C-suite meetings; and
- avoid a narrow focus on controls or certain risks—cybersecurity demands a holistic approach.
Chief information officers/chief information security officers should:
- get security operations (SecOps) leaders to validate generative AI output, particularly threat-detection algorithms updated by generative AI;
- train new and junior SecOps employees to hunt threats with and without generative AI to avoid dependence; and
- where possible, avoid relying on a single vendor or generative AI model across the cybersecurity stack.
Cybersecurity companies should:
- hire the right mix of talent to bring generative AI capabilities into their products; and
- guard against generative AI–created false information (hallucinations) and external tampering with generative AI algorithms and models that might create backdoor vulnerabilities.
Generative AI will rapidly advance, and it’s essential that all stakeholders from cybersecurity providers to enterprises continuously update their specialist knowledge and strategy to take advantage—and stay protected.
Este artigo faz parte do Relatório de Fusões e Aquisições de 2023 da Bain.